The 2024 Nobel Prize in chemistry acknowledged Demis Hassabis, John Jumper and David Baker for utilizing machine studying to deal with one in every of biology’s largest challenges: predicting the 3D form of proteins and designing them from scratch.
This 12 months’s award stood out as a result of it honored analysis that originated at a tech firm: DeepMind, an AI analysis startup that was acquired by Google in 2014. Most earlier chemistry Nobel Prizes have gone to researchers in academia.
Many laureates went on to kind startup firms to additional broaden and commercialize their groundbreaking work – as an illustration, CRISPR gene-editing know-how and quantum dots – however the analysis, from begin to finish, wasn’t accomplished within the industrial sphere.
Though the Nobel Prizes in physics and chemistry are awarded individually, there’s a fascinating connection between the profitable analysis in these fields in 2024.
The physics award went to 2 laptop scientists who laid the foundations for machine studying, whereas the chemistry laureates had been rewarded for his or her use of machine studying to deal with one in every of biology’s largest mysteries: how proteins fold.
The 2024 Nobel Prizes underscore each the significance of this sort of synthetic intelligence and the way science in the present day typically crosses conventional boundaries, mixing completely different fields to attain groundbreaking outcomes.
The problem of protein folding
Proteins are the molecular machines of life. They make up a good portion of our our bodies, together with muscle mass, enzymes, hormones, blood, hair and cartilage.
(©Johan Jarnestad/The Royal Swedish Academy of Sciences)
Understanding proteins’ buildings is important as a result of their shapes decide their features.
Again in 1972, Christian Anfinsen received the Nobel Prize in chemistry for displaying that the sequence of a protein’s amino acid constructing blocks dictates the protein’s form, which, in flip, influences its perform. If a protein folds incorrectly, it might not work correctly and will result in ailments reminiscent of Alzheimer’s, cystic fibrosis or diabetes.
A protein’s general form relies on the tiny interactions, the sights and repulsions, between all of the atoms within the amino acids it is product of. Some wish to be collectively, some do not. The protein twists and folds itself right into a remaining form primarily based on many hundreds of those chemical interactions.
For many years, one in every of biology’s biggest challenges was predicting a protein’s form primarily based solely on its amino acid sequence.
Though researchers can now predict the form, we nonetheless do not perceive how the proteins maneuver into their particular shapes and decrease the repulsions of all of the interatomic interactions in a number of microseconds.
To know how proteins work and to forestall misfolding, scientists wanted a solution to predict the best way proteins fold, however fixing this puzzle was no simple job.
In 2003, College of Washington biochemist David Baker wrote Rosetta, a pc program for designing proteins. With it he confirmed it was attainable to reverse the protein-folding drawback by designing a protein form after which predicting the amino acid sequence wanted to create it.
It was an outstanding soar ahead, however the form chosen for the calculation was easy, and the calculations had been advanced. A significant paradigm shift was required to routinely design novel proteins with desired buildings.
A brand new period of machine studying
Machine studying is a sort of AI the place computer systems study to unravel issues by analyzing huge quantities of knowledge. It has been utilized in numerous fields, from game-playing and speech recognition to autonomous automobiles and scientific analysis.
The concept behind machine studying is to make use of hidden patterns in information to reply advanced questions.
This method made an enormous leap in 2010 when Demis Hassabis co-founded DeepMind, an organization aiming to mix neuroscience with AI to unravel real-world issues.
Hassabis, a chess prodigy at age 4, rapidly made headlines with AlphaZero, an AI that taught itself to play chess at a superhuman degree. In 2017, AlphaZero completely beat the world’s prime laptop chess program, Stockfish-8.
The AI’s capability to study from its personal gameplay, relatively than counting on preprogrammed methods, marked a turning level within the AI world.
Quickly after, DeepMind utilized related strategies to Go, an historical board recreation recognized for its immense complexity. In 2016, its AI program AlphaGo defeated one of many world’s prime gamers, Lee Sedol, in a broadly watched match that surprised tens of millions.
In 2016, Hassabis shifted DeepMind’s focus to a brand new problem: the protein-folding drawback. Below the management of John Jumper, a chemist with a background in protein science, the AlphaFold challenge started.
The group used a big database of experimentally decided protein buildings to coach the AI, which allowed it to study the ideas of protein folding.
The end result was AlphaFold2, an AI that might predict the 3D construction of proteins from their amino acid sequences with exceptional accuracy.
This was a big scientific breakthrough. AlphaFold has since predicted the buildings of over 200 million proteins – primarily all of the proteins that scientists have sequenced thus far. This large database of protein buildings is now freely accessible, accelerating analysis in biology, medication and drug growth.
Designer proteins to combat illness
Understanding how proteins fold and performance is essential for designing new medication. Enzymes, a sort of protein, act as catalysts in biochemical reactions and might velocity up or regulate these processes.
To deal with ailments reminiscent of most cancers or diabetes, researchers typically goal particular enzymes concerned in illness pathways. By predicting the form of a protein, scientists can determine the place small molecules – potential drug candidates – would possibly bind to it, which is step one in designing new medicines.
In 2024, DeepMind launched AlphaFold3, an upgraded model of the AlphaFold program that not solely predicts protein shapes but in addition identifies potential binding websites for small molecules. This advance makes it simpler for researchers to design medication that exactly goal the suitable proteins.
Google purchased Deepmind for reportedly round half a billion {dollars} in 2014. Google DeepMind has now began a brand new enterprise, Isomorphic Labs, to collaborate with pharmaceutical firms on real-world drug growth utilizing these AlphaFold3 predictions.
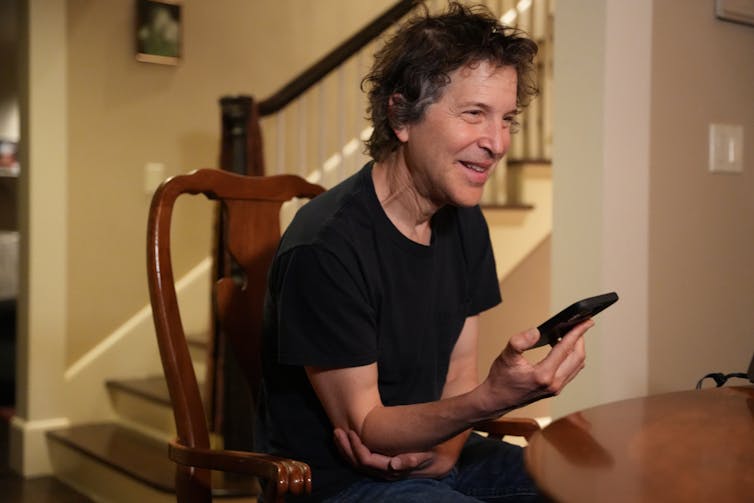
For his half, David Baker has continued to make important contributions to protein science. His group on the College of Washington developed an AI-based technique known as “family-wide hallucination,” which they used to design solely new proteins from scratch.
Hallucinations are new patterns – on this case, proteins – which can be believable, that means they’re a superb match with patterns within the AI’s coaching information.
These new proteins included a light-emitting enzyme, demonstrating that machine studying will help create novel artificial proteins. These AI instruments provide new methods to design useful enzymes and different proteins that by no means may have developed naturally.
AI will allow analysis’s subsequent chapter
The Nobel-worthy achievements of Hassabis, Jumper and Baker present that machine studying is not only a device for laptop scientists – it is now an important a part of the way forward for biology and medication.
By tackling one of many hardest issues in biology, the winners of the 2024 prize have opened up new prospects in drug discovery, personalised medication and even our understanding of the chemistry of life itself.
Marc Zimmer, Professor of Chemistry, Connecticut Faculty
This text is republished from The Dialog below a Artistic Commons license. Learn the unique article.