In case your jaw dropped as you watched the most recent AI-generated video, your financial institution steadiness was saved from criminals by a fraud detection system, or your day was made somewhat simpler since you have been capable of dictate a textual content message on the run, you have got many scientists, mathematicians and engineers to thank.
However two names stand out for foundational contributions to the deep studying expertise that makes these experiences potential: Princeton College physicist John Hopfield and College of Toronto laptop scientist Geoffrey Hinton.
The 2 researchers have been awarded the Nobel Prize in physics on Oct. 8, 2024, for his or her pioneering work within the discipline of synthetic neural networks.
Although synthetic neural networks are modeled on organic neural networks, each researchers’ work drew on statistical physics, therefore the prize in physics.
How a neuron computes
Synthetic neural networks owe their origins to research of organic neurons in residing brains. In 1943, neurophysiologist Warren McCulloch and logician Walter Pitts proposed a easy mannequin of how a neuron works.
Within the McCulloch-Pitts mannequin, a neuron is related to its neighboring neurons and might obtain indicators from them. It may possibly then mix these indicators to ship indicators to different neurons.
However there’s a twist: It may possibly weigh indicators coming from completely different neighbors otherwise. Think about that you’re making an attempt to resolve whether or not to purchase a brand new bestselling cellphone. You discuss to your mates and ask them for his or her suggestions.
A easy technique is to gather all buddy suggestions and resolve to associate with regardless of the majority says. For instance, you ask three associates, Alice, Bob and Charlie, they usually say yay, yay and nay, respectively. This leads you to a call to purchase the cellphone as a result of you have got two yays and one nay.
Nevertheless, you would possibly belief some associates extra as a result of they’ve in-depth information of technical devices. So that you would possibly resolve to provide extra weight to their suggestions.
For instance, if Charlie could be very educated, you would possibly depend his nay thrice and now your choice is to not purchase the cellphone – two yays and three nays.
For those who’re unlucky to have a buddy whom you utterly mistrust in technical gadget issues, you would possibly even assign them a unfavorable weight. So their yay counts as a nay and their nay counts as a yay.
As soon as you have made your personal choice about whether or not the brand new cellphone is an efficient selection, different associates can ask you on your suggestion.
Equally, in synthetic and organic neural networks, neurons can combination indicators from their neighbors and ship a sign to different neurons.
This functionality results in a key distinction: Is there a cycle within the community? For instance, if I ask Alice, Bob and Charlie at present, and tomorrow Alice asks me for my suggestion, then there’s a cycle: from Alice to me, and from me again to Alice.
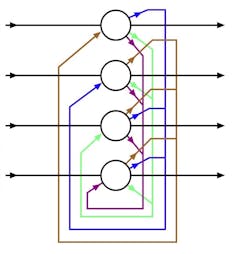
(Zawersh/Wikimedia, CC BY-SA)
If the connections between neurons would not have a cycle, then laptop scientists name it a feedforward neural community. The neurons in a feedforward community will be organized in layers.
The primary layer consists of the inputs. The second layer receives its indicators from the primary layer and so forth. The final layer represents the outputs of the community.
Nevertheless, if there’s a cycle within the community, laptop scientists name it a recurrent neural community, and the preparations of neurons will be extra difficult than in feedforward neural networks.
Hopfield community
The preliminary inspiration for synthetic neural networks got here from biology, however quickly different fields began to form their improvement. These included logic, arithmetic and physics.
The physicist John Hopfield used concepts from physics to review a specific kind of recurrent neural community, now referred to as the Hopfield community. Specifically, he studied their dynamics: What occurs to the community over time?
Such dynamics are additionally necessary when data spreads by way of social networks. Everybody’s conscious of memes going viral and echo chambers forming in on-line social networks. These are all collective phenomena that in the end come up from easy data exchanges between folks within the community.
Hopfield was a pioneer in utilizing fashions from physics, particularly these developed to review magnetism, to know the dynamics of recurrent neural networks. He additionally confirmed that their dynamics can give such neural networks a type of reminiscence.
Boltzmann machines and backpropagation
Throughout the Nineteen Eighties, Geoffrey Hinton, computational neurobiologist Terrence Sejnowski and others prolonged Hopfield’s concepts to create a brand new class of fashions referred to as Boltzmann machines, named for the Nineteenth-century physicist Ludwig Boltzmann.
Because the identify implies, the design of those fashions is rooted within the statistical physics pioneered by Boltzmann.
Not like Hopfield networks that would retailer patterns and proper errors in patterns – like a spellchecker does – Boltzmann machines may generate new patterns, thereby planting the seeds of the fashionable generative AI revolution.
Hinton was additionally a part of one other breakthrough that occurred within the Nineteen Eighties: backpropagation. In order for you synthetic neural networks to do fascinating duties, it’s a must to by some means select the best weights for the connections between synthetic neurons.
Backpropagation is a key algorithm that makes it potential to pick out weights primarily based on the efficiency of the community on a coaching dataset. Nevertheless, it remained difficult to coach synthetic neural networks with many layers.
Within the 2000s, Hinton and his co-workers cleverly used Boltzmann machines to coach multilayer networks by first pretraining the community layer by layer after which utilizing one other fine-tuning algorithm on prime of the pretrained community to additional modify the weights.
Multilayered networks have been rechristened deep networks, and the deep studying revolution had begun.
allowfullscreen=”allowfullscreen” frameborder=”0″>
AI pays it back to physics
The Nobel Prize in physics shows how ideas from physics contributed to the rise of deep learning. Now deep learning has begun to pay its due back to physics by enabling accurate and fast simulations of systems ranging from molecules and materials all the way to the entire Earth’s climate.
By awarding the Nobel Prize in physics to Hopfield and Hinton, the prize committee has signaled its hope in humanity’s potential to use these advances to promote human well-being and to build a sustainable world.
Ambuj Tewari, Professor of Statistics, College of Michigan
This text is republished from The Dialog below a Artistic Commons license. Learn the unique article.