“One Guinness, please!” says a buyer to a barkeep, who flips a branded pint glass and catches it below the faucet. The barkeep begins a multistep pour course of lasting exactly 119.5 seconds, which, whether or not it’s a advertising gimmick or a marvel of alcoholic engineering, has turn into a beloved ritual in Irish pubs worldwide. The outcome: a wealthy stout with an ideal froth layer like an earthy milkshake.
The Guinness brewery has been identified for revolutionary strategies ever since founder Arthur Guinness signed a 9,000-year lease in Dublin for £45 a yr. For instance, a mathematician-turned-brewer invented a chemical approach there after 4 years of tinkering that offers the brewery’s namesake stout its velvety head. The strategy, which entails including nitrogen fuel to kegs and to little balls inside cans of Guinness, led to at present’s massively well-liked “nitro” brews for beer and espresso.
However essentially the most influential innovation to come back out of the brewery by far has nothing to do with beer. It was the birthplace of the t-test, one of the crucial essential statistical methods in all of science. When scientists declare their findings “statistically significant,” they fairly often use a t-test to make that dedication. How does this work, and why did it originate in beer brewing, of all locations?
On supporting science journalism
If you happen to’re having fun with this text, think about supporting our award-winning journalism by subscribing. By buying a subscription you might be serving to to make sure the way forward for impactful tales concerning the discoveries and concepts shaping our world at present.
Close to the beginning of the twentieth century, Guinness had been in operation for nearly 150 years and towered over its rivals because the world’s largest brewery. Till then, high quality management on its merchandise consisted of tough eyeballing and scent assessments. However the calls for of world enlargement motivated Guinness leaders to revamp their method to focus on consistency and industrial-grade rigor. The corporate employed a staff of brainiacs and gave them latitude to pursue analysis questions in service of the right brew. The brewery turned a hub of experimentation to reply an array of questions: The place do the finest barley varieties develop? What’s the excellent saccharine degree in malt extract? How a lot did the most recent advert marketing campaign enhance gross sales?
Amid the flurry of scientific power, the staff confronted a persistent drawback: deciphering its information within the face of small pattern sizes. One problem the brewers confronted entails hop flowers, important components in Guinness that impart a bitter taste and act as a pure preservative. To evaluate the standard of hops, brewers measured the gentle resin content material within the vegetation. Let’s say they deemed 8 % an excellent and typical worth. Testing each flower within the crop wasn’t economically viable, nevertheless. So that they did what any good scientist would do and examined random samples of flowers.
Let’s examine a made-up instance. Suppose we measure gentle resin content material in 9 samples and, as a result of samples differ, observe a spread of values from 4 % to 10 %, with a median of 6 %—too low. Does that imply we should always dump the crop? Uncertainty creeps in from two potential explanations for the low measurements. Both the crop actually does comprise unusually low gentle resin content material, or although the samples comprise low ranges, the complete crop is definitely wonderful. The entire level of taking random samples is to depend on them as devoted representatives of the complete crop, however maybe we had been unfortunate by selecting samples with uncharacteristically low ranges. (We solely examined 9, in spite of everything.) In different phrases, ought to we think about the low ranges in our samples considerably totally different from 8 % or mere pure variation?
This quandary is just not distinctive to brewing. Moderately, it pervades all scientific inquiry. Suppose that in a medical trial, each the therapy group and placebo group enhance, however the therapy group fares just a little higher. Does that present enough grounds to advocate the remedy? What if I instructed you that each teams truly obtained two totally different placebos? Would you be tempted to conclude that the placebo within the group with higher outcomes should have medicinal properties? Or might it’s that whenever you observe a bunch of individuals, a few of them will simply naturally enhance, typically by just a little and typically by lots? Once more, this boils all the way down to a query of statistical significance.
The idea underlying these perennial questions within the area of small pattern sizes hadn’t been developed till Guinness got here on the scene—particularly, not till William Sealy Gosset, head experimental brewer at Guinness within the early twentieth century, invented the t-test. The idea of statistical significance predated Gosset, however prior statisticians labored within the regime of huge pattern sizes. To understand why this distinction issues, we have to perceive how one would decide statistical significance.
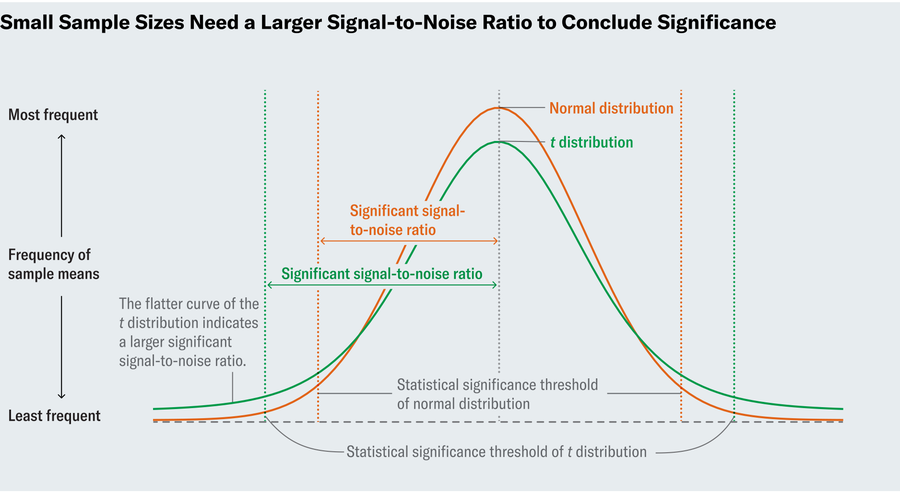
Keep in mind, the hops samples in our state of affairs have a median gentle resin content material of 6 %, and we wish to know whether or not the common within the full crop truly differs from the specified 8 % or if we simply obtained unfortunate with our pattern. So we’ll ask the query: What’s the chance that we might observe such an excessive worth (6 %) if the complete crop was in reality typical (with a median of 8 %)?Historically, if this chance, referred to as a P worth, lies beneath 0.05, then we deem the deviation statistically vital, though totally different functions name for various thresholds.
Usually two separate components have an effect on the P worth: how far a pattern deviates from what is predicted in a inhabitants and the way frequent massive deviations are. Consider this as a tug-of-war between sign and noise. The distinction between our noticed imply (6 %) and our desired one (8 %) offers the sign—the bigger this distinction, the extra seemingly the crop actually does have low gentle resin content material. The usual deviation amongst flowers brings the noise. Normal deviation measures how unfold out the info are across the imply; small values point out that the info hover close to the imply, and bigger values indicate wider variation. If the gentle resin content material usually fluctuates extensively throughout buds (in different phrases, has a excessive normal deviation), then perhaps the 6 % common in our pattern shouldn’t concern us. But when flowers are inclined to exhibit consistency (or a low normal deviation), then 6 % might point out a real deviation from the specified 8 %.
To find out a P worth in a really perfect world, we’d begin by calculating the signal-to-noise ratio. The upper this ratio, the extra confidence we now have within the significance of our findings as a result of a excessive ratio signifies that we’ve discovered a real deviation. However what counts as excessive signal-to-noise? To deem 6 % considerably totally different from 8 %, we particularly wish to know when the signal-to-noise ratio is so excessive that it solely has a 5 % likelihood of occurring in a world the place an 8 % resin content material is the norm. Statisticians in Gosset’s time knew that if you happen to had been to run an experiment many occasions, calculate the signal-to-noise ratio in every of these experiments and graph the outcomes, that plot would resemble a “standard normal distribution”—the acquainted bell curve. As a result of the conventional distribution is properly understood and documented, you possibly can lookup in a desk how giant the ratio have to be to succeed in the 5 % threshold (or another threshold).
Gosset acknowledged that this method solely labored with giant pattern sizes, whereas small samples of hops wouldn’t assure that standard distribution. So he meticulously tabulated new distributions for smaller pattern sizes. Now often known as t-distributions, these plots resemble the conventional distribution in that they’re bell-shaped, however the curves of the bell don’t drop off as sharply. That interprets to needing an excellent bigger signal-to-noise ratio to conclude significance. His t-test permits us to make inferences in settings the place we couldn’t earlier than.
Mathematical marketing consultant John D. Cook dinner mused on his weblog in 2008 that maybe it mustn’t shock us that the t-test originated at a brewery versus, say, a vineyard. Brewers demand consistency of their product, whereas vintners experience selection. Wines have “good years,” and every bottle tells a narrative, however you need each pour of Guinness to ship the identical trademark style. On this case, uniformity impressed innovation.
Gosset solved many issues on the brewery along with his new approach. The self-taught statistician revealed his t-test below the pseudonym “Student” as a result of Guinness didn’t wish to tip off rivals to its analysis. Though Gosset pioneered industrial high quality management and contributed a great deal of different concepts to quantitative analysis, most textbooks nonetheless name his nice achievement the “Student’s t-test.” Historical past might have uncared for his identify, however he may very well be proud that the t-test is without doubt one of the most generally used statistical instruments in science to this present day. Maybe his accomplishment belongs in Guinness World Information (the thought for which was dreamed up by Guinness’s managing director within the Fifties). Cheers to that.